Use Industrial AI to model, simulate, and deploy Transactive Energy solutions.
As an emerging market-based technique for managing power generation and consumption, Transactive Energy requires new software architectures.
Balancing power supply and demand in real time within the distribution grid is increasingly difficult due to rapid emergence of decentralized and variable generation resources, such as wind, solar, and cogeneration. Transactive Energy (TE) is an emerging set of techniques that applies market principles for managing this balance. At its core, TE can be thought of as a framework for dynamic pricing of power, similar in concept to how airlines dynamically price their seats to best match supply and demand.
TE concepts are already in use for the transmission grid via wholesale power markets. To bring TE to distribution will require new regulations, business models, and architectures for the software systems that model, simulate, and deploy TE.
Use Case Problem:
Solution:
A SmartCloud application demonstrates the value of Transactive Energy's market pricing techniques in balancing a transmission grid. The demonstration simulates wholesale power market behavior in response to varying grid and weather conditions.
Data needed to feed simulations is modeled and integrated using the electric industry's Common Information Model (CIM) standard and includes: grid assets such as generators, power lines, and substations; weather feeds from commercially available sources; and market participant roles including buyers and sellers.
The application integrates third-party systems including ESRI ArcGIS for map visualizations, Microsoft PowerBI for data plots, Microsoft Events Hub for large scale data ingestion, Microsoft Stream Analytics for detecting events of interest.
AI Agents acting on behalf of each market participant simulate buy and sell decisions. For example:
-
agents for generators bid to sell power at various times with the goal of maximizing profits;
-
agents for buyers, such as Load Serving Entities, bid to buy with the goal of ensuring supply for consumers at the lowest possible prices; and
-
grid operator agents matched buy/sell bids with the goal of ensuring efficient and reliable operation of the grid.
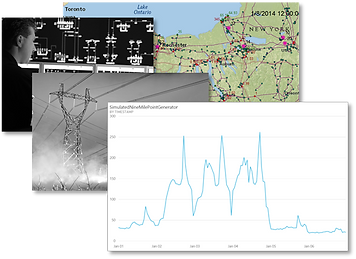
Results:
-
AI Agents for modeling and simulation: Use AI Agents to act autonomously on behalf of each TE participant for realistic simulation of TE systems. For example, agents modeling and simulating buyers would continuously bid to buy electricity at prices for specified times while other agents for sellers would bid to sell power. Market manager Agents would match the buy/sell bids in a way that optimally meets supply/demand while preventing violation of any physical constraints for the grid.
-
AI Agents for deployment: Assign each TE participant a configurable AI Agent acting on their behalf in a market in real time. As bids are accepted market manager agents communicate actions to other systems such as the grid operator's advanced distribution management system or billing system.
-
Situational Management: Use Industrial AI technologies for real-time solutions that improve overall performance and reliability of TE-driven grids, including solutions that deliver situational awareness, predictive alerts, causal analysis, simulations, optimizations, and supervisory control.
-
Auditable data: Permanently store corrected and uncorrected data to support audits of TE transactions and offline analytics.
-
Hybrid Cloud / SaaS: Reduce overall solution costs by optionally deploying parts of the solution in the cloud using a software-as-a-service model.
Technologies:
Improved design of Transactive Energy systems and better monitoring and auditing of deployed systems.
Transactive Energy for Balancing the Grid
Home >> Solutions >> Energy and Utilities >> Transactive Energy Use Case
© SmartCloud, Inc., All rights reserved.