Let's Make a Deal with Bayes
You are on a famous game show with three doors to choose from, each
hiding a prize. One will make you very wealthy while the others are
silly, worthless jokes…
You pick the middle door. But then to heighten the drama, the show host,
who knows what is behind each door, opens the one on the left, which is
worthless, and offers you a deal to either keep your first choice or switch.
What should you do? Stay in the middle or switch to the right? Which
door gives the best chance for wealth? Or does it not matter?
March 31, 2016

Simple but powerful
The theorem is based on a fairly simple, but powerful equation:
P (H|E) = P(E|H) P(H) / P(E)
Which translates to:
(Probability of Hypotheses H being true given that Evidence E occurs)
=
(Probability of E being true given that H occurs) *
(Probability of H occurring by itself) / (Probability of E occurring by itself)
For those of you that are mathematically inclined, there are many good sources for
learning how the theorem works and how to apply it, such as to our game show
scenario. Here is a succinct and very understandable one-minute visualization
of Bayes theorem. And here is a helpful ten-minute video that walks through the
Bayesian logic behind the door selection decision, which has become known
as the Monty Hall problem in honor of the original host of the
“Let’s Make A Deal” game show.

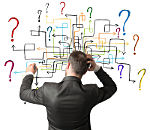

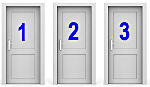

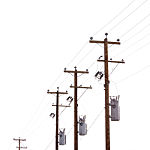

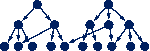
Bayes to the rescue
The path to your answer starts with Reverend Thomas Bayes from the 18th century. He created the equation, now known as Bayes theorem, that represents the probability of an event as new information is learned.
In our game show case, the first event is choosing between three
doors. Of course the probability of selecting the door with wealth is
one out of three, or 33 percent. Similarly, the probability of that
door being among the other unselected doors is two out of three,
or 66 percent.
The second event, our host opening one of the worthless doors,
adds important new information for assessing the probability of
wealth behind each of the two remaining doors: your original
selection (middle) and the one you could switch to (right).
Bayes theorem tells us that with this new information we now know that the probability of the remaining unselected door leading to wealth is two out of three. Our original middle door only has a probability of success of one out of three.
If you want the best chance for wealth, you should switch!
Many might at first think the odds are one out of two for either of the remaining doors. But a key insight with Bayes is the need to continually update the probabilities of an event as new information is learned. This insight, in combination with recent advancements in computing power and software tools, have made Bayesian techniques a top choice for reasoning about uncertain situations within artificial intelligence solutions.
Managing situations with Bayes
SmartCloud is embracing Bayes theorem in the form of Bayesian belief networks
as an integral part of our Situational Management solutions. For example we are
developing use cases for assessing risky situations within utility grids and for fraud
detection.
Bayesian networks fit very well with how we represent the knowledge needed to reason about a situation, in particular for the very common case of evidence missing or being highly uncertain. Bayesian networks also learn from new data by adapting the probabilities assigned between related events.
With Bayesian networks we model the probability of a situational state being true as the values of its various contributing factors become known. The modelling is based on a combination of historical analysis and human expertise. An important advantage of this approach is the ability to explain the causes of a situation to humans, helping them to more effectively decide on a response.
level of uncertainty – just like we did when selecting the first door in our game show. We would start a Bayesian network analysis for our grid by assuming a base probability of imbalance problems – say 3% for this scenario. (The base probability in the game show was 33%).
As we learn more information, we traverse our network to update the probability. For instance if we learn that the weather will have record high temperatures during the day, creating high electric consumption for air conditioning, we might recalculate the probability to be 20%. Adding the information that it is a weekday, Wednesday, typically a high consumption day, might increase the probability to 30%. Then considering it is 6 pm, usually the peak of a day, brings the odds to 40%. If a major regional generator is out of service due to maintenance issues, then the odds might jump to 60%. And finally if the weather is to be very calm all day, meaning that wind generated power will be unavailable, then the odds jump to 75%.
Knowing that the odds of an electrical imbalance situation are predicted to be high and what the major causes are guides decision making and actions for avoiding its impacts. Utility operators might, for instance, request customers to curtail electric use as part of a demand response program to mitigate peak consumption times and/or increase their bids to purchase electricity from the energy spot market to make up for unavailable generation.
Power grid use case
As an example of a Bayesian use case, the likelihood that a utility’s electric grid
will have a problematic energy imbalance situation in the days to come is
dependent on many factors. These might include factors related to consumption
like weather, time of the day, or day of the week, together with those related to
supply like available generation and hourly electricity prices on the wholesale
spot market.
If we do not know the values for any these factors, then we are facing the greatest
Your situation is one of uncertainty. First there is the uncertainty of which the three doors to pick and then, second, the uncertainty between the final two doors.
It turns out that the path to your best answer is on the same path that SmartCloud is using for managing situations within complex business and industrial operations, like for risks within utility grids or tax fraud in retail networks.
Where are your uncertainties?
At SmartCloud we are evolving state-of-the-art AI techniques for applying Bayesian analysis to management of real-time situations for energy, oil and gas, process manufacturing, and fraud.
We’re combining the advantages of Bayes theorem, semantic modelling, rules, and other AI reasoning technologies to augment the ability of humans to reach useful conclusions when they are facing high levels of uncertainty. We’d welcome a discussion about your operational situations of interest, risks, opportunities, and uncertainties so that we can together evaluate a modern application of Reverend Bayes’s insights.
© SmartCloud, Inc., All rights reserved.